-
Digital Natives
Unlock growth with Grant Thornton Bharat's Digital Natives solutions. Customised support for tech-driven companies in healthcare, gaming, and more.
-
Business Consulting
Our business consulting specialists offer a comprehensive blend of strategic advisory services. We assess the business, industry, operating model, synergy, skill sets and vision of the organisation and recommend the way forward
-
Digital Transformation Services
Grant Thornton’s digital transformation services help traditional businesses digitalise their business models with cloud technology, IoT consulting, app development and more DigiTech solutions.
-
Human Capital Consulting
Our Human Capital Consulting team harnesses technology and industry expertise to assist in constructing adaptable organisations with transparency, fostering productive and value-driven workforces, and inspiring employees to engage meaningfully in their tasks.
-
Production Linked Incentive Scheme
Production-linked Incentive Scheme by the Indian government is aimed at boosting manufacturing. Grant Thornton Bharat offers varied services across sectors to help businesses avail of this scheme.
-
Public Sector Advisory
Our Public Sector Advisory team has focused streams, aligned with the core priorities of the Government of India. We are responsible for providing innovative and customized technical and managerial solutions.
-
Tech Advisory
We have amalgamated Digital Transformation, IT Advisory & Information Management and Analytics into a new offering, DigiTech.
-
Direct Tax services
Our tax specialists offer a comprehensive blend of tax services, tax litigation, regulatory and compliance services, helping you navigate through complex business matters.
-
Indirect Tax Services
Get tax services by leading tax firm Grant Thornton India. Our indirect tax services include consulting, compliance and litigation services for corporate, international and transaction tax
-
Transfer pricing services
Our transfer pricing services experts provide a range of services from provision of APA services to handling large global assignments including Country by Country reporting.
-
US Tax
At Grant Thornton, we help individuals and dynamic companies deal with US tax laws, which are one of the most complicated tax legislations across the world.
-
Financial Services - Tax
Best financial advisory services, tailored for small and large businesses by the experts having comprehensive knowledge of domestic laws and access to multifaceted tools to provide a valuable results.
-
Financial Reporting consulting services
Our experts have significant hands-on experience in providing IFRS/US GAAP services, end-to-end solutions and support services to fulfil financial reporting requirements.
-
Fund accounting and financial reporting
International operations often lack standardisation and have varied local reporting formats and requirements. Our experts can offer proactive insights, practical guidance, and positive progress and help meet regulatory timeframes.
-
Compliance and Secretarial Services
Our experts can assist in overhauling the entire compliance machinery of the organisation through evaluation of the applicable statutory obligations, monitoring of adequate governance controls, reporting and providing ongoing support.
-
Global People Solutions
As businesses transcend borders, both domestic and global considerations need equal attention. Our interim CFO and financial controller support services help organisations meet the business vision.
-
Finance and accounting outsourcing
Our accounting experts assist organisations in managing their accounting and reporting. Our dedicated Integrated Knowledge and Capability Centre (IKCC), allows us to service both the domestic and global markets efficiently and cost-effectively
-
Compliance Management System
We have automation solutions for you that will allow meeting government requirements and remain diligent, which when failed, can lead to penalties and loss in revenue.
-
IKCC: Grant Thornton's Shared Service Centre
The India Knowledge and Capability Centre (IKCC), aimed at delivering solutions by developing capabilities, has completed four years of its journey.
-
Global compliance and reporting solutions
At Grant Thornton Bharat, we meet the challenges of our clients and help them unlock their potential for growth. Our professionals offer solutions tailored to meet our clients’ global accounting and statutory reporting requirements. With first-hand experience of local reporting requirements in more than 145+ locations worldwide, we provide seamless and consistent international service delivery through a single point of contact.
-
Related Party Transactions Governance
Grant Thornton Bharat's comprehensive related-party transaction services ensure good governance by adhering to regulatory requirements, promoting transparency, and providing robust policies for compliance, documentation, and accountability in related-party transactions.
-
Private Client Services
Grant Thornton Bharat Private Client Services offers tailored advisory for family-owned businesses, focusing on governance, compliance, tax, succession planning, and family office structuring to sustain wealth and preserve legacies across generations.
-
GTMitra: Tax & Regulatory Tool
GTMitra, a specialised tax and regulatory tool by Grant Thornton Bharat, supports multinational businesses in understanding laws and regulations for effective growth strategies.
-
Labour codes
Labour codes solutions help you transition through the new legislation. At Grant Thornton, we help businesses divide their approach to make sure a smooth transition.
-
Alerts
At Grant Thornton India, with the help of our tax alerts, we help to provide updates on how to minimise your tax exposure and risks.
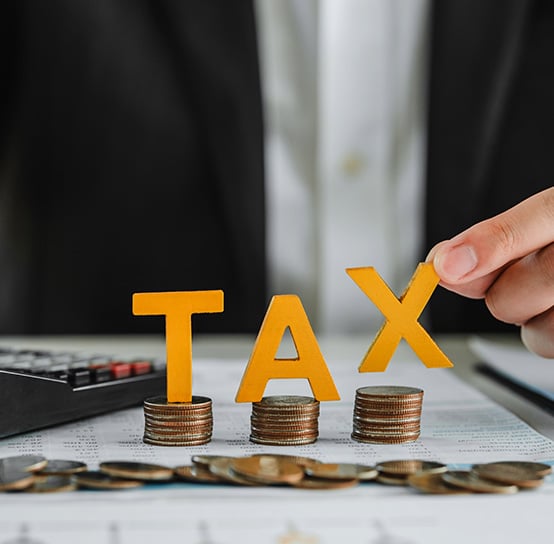
-
Cyber
In today’s time, businesses have gone through large transformation initiatives such as adoption of digital technologies, transition to cloud, use of advanced technologies et al.
-
Governance, Risk & Operations
Our Governance, Risk and Operations (GRO) services encompass Internal Audit, Enterprise Risk Management, Internal Financial Controls, IT advisory, Standard Operating Procedures and other services.
-
Risk analytics
Grant Thornton Bharat’s CLEARR Insights is a state-of-the art data analytics platform that will help you in seamless data analysis and efficient decision-making.
-
Forensic & Investigation Services
The team of forensic advisory services experts consists of the best intelligence corporate experts, and fraud risk, computer forensic experts to deliver most effective solutions to dynamic Indian businesses.
-
ESG consulting
Grant Thornton Bharat offers holistic ESG consulting solutions for sustainable business outcomes. With industry expertise and AI technology, we drive long-term value.
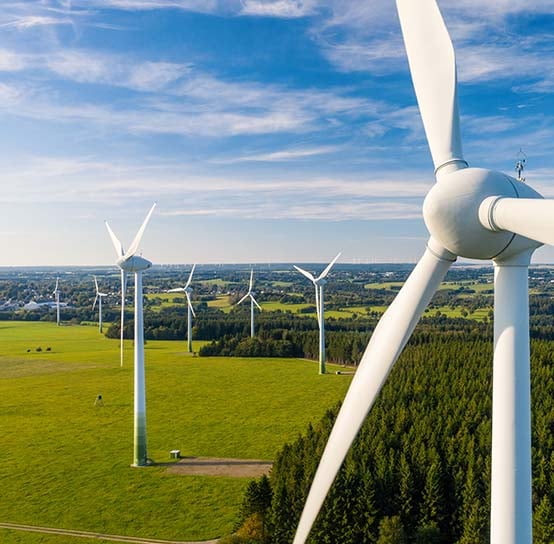
-
Transaction Tax Services
Our transaction tax experts understand your business, anticipate your needs and come up with robust tax solutions that help you achieve business objectives ensuring compliance and efficiency
-
Deal Advisory
Unlike other M&A advisory firm in India, we offer deal advisory services and work exclusively with controlled and well-designed strategies to help businesses grow, expand and create value.
-
Due Diligence
Grant Thornton’s financial due diligence services are aimed at corporate looking for mergers and acquisitions, private equity firms evaluating investments and businesses/promoters considering sale/divestment.
-
Valuations
As one of the leading valuation consultants in India, Grant Thornton specializes in all the aspects of the process like business valuation services, financial reporting, tax issues, etc.
-
Overseas Listing
Overseas listing presents a perfect platform for mid-sized Indian companies with global ambitions. Grant Thornton’s team of experts in listings, work closely with clients during all stages.
-
Debt & Special Situations Solutions
Grant Thornton Bharat offers specialist debt and special situations consulting services, including restructuring, insolvency, and asset tracing solutions.
-
Financial Reporting Advisory Services
Grant Thornton Bharat Financial Reporting Advisory Services offer end-to-end solutions for complex financial requirements, including GAAP conversions, IPO support, and hedge accounting advisory, ensuring accurate financial reporting and compliance.
-
Financial Statement Audit and Attestation Services
Grant Thornton Bharat offers customised financial statement audit and attestation services, ensuring impeccable quality and compliance with global standards. Our partner-led approach, technical expertise, and market credibility ensure effective solutions for your business needs.
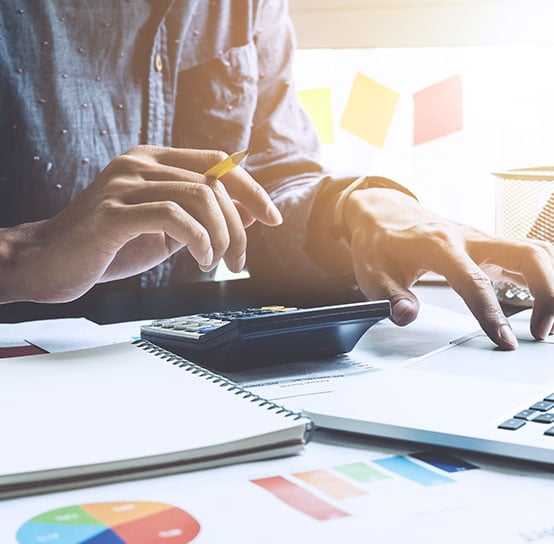
- Agriculture
- Asset management
- Automotive and EV
- Aviation
- Banking
- Education and ed-tech
- Energy & Renewables
- Engineering & industrial products
- FinTech
- FMCG & consumer goods
- Food processing
- Gaming
- Healthcare
- Urban infrastructure
- Insurance
- Media
- Medical devices
- Metals & Mining
- NBFC
- Pharma, bio tech & life sciences
- Real estate and REITs
- Retail & E-commerce
- Specialty chemicals
- Sports
- Technology
- Telecom
- Transportation & logistics
- Tourism & hospitality
-
Article Improving credit penetration in BiharRBI’s priority sector lending guidelines have a key role to play in providing credit to small farmers, artisans and micro firms
-
Article Sustainable farming and its economic imperativeSustainable farming in India is crucial for food security, economic growth, and environmental conservation, requiring government support and farmer education.
-
Article Agriculture and Budget: Immediate compulsions and long-term visionGovernment focuses on sustainable agriculture, digital infrastructure, and market intelligence to enhance productivity and global competitiveness in agriculture.
-
Article Union Budget 2024 expectations: Building resilience for consumer industryUnion Budget 2024 expectations: Building resilience for consumer industry
-
India-UK
India-UK
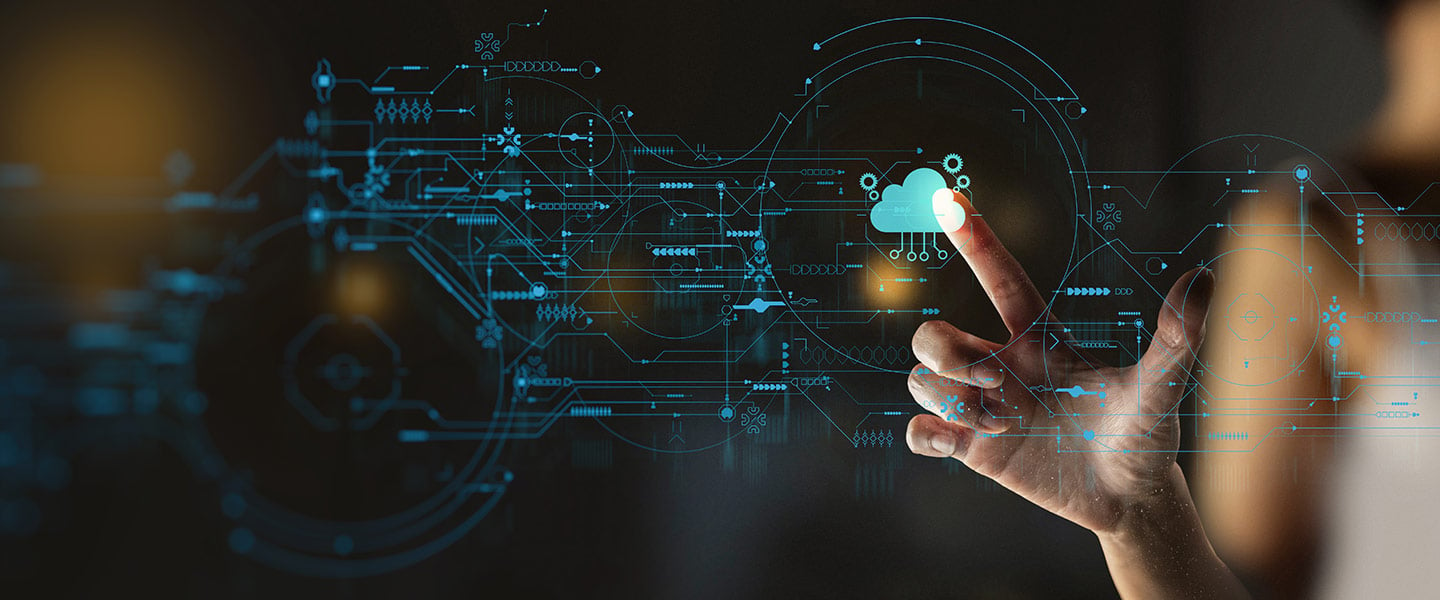
In an era where data is central to business strategy, migrating data to the cloud has become a transformative move for organisations aiming to harness its full potential. Cloud migration is not just about shifting storage from on-premises systems to a cloud environment, it is about revolutionising how businesses analyse, process, and utilise their data. This blog explores the opportunities, benefits, challenges, and future trends associated with data migration to the cloud, focusing on enhancing analytics capabilities.
Opportunities in cloud data migration
- Advanced analytical capabilities
One of the most significant opportunities cloud migration presents is leveraging advanced analytics tools. Cloud platforms such as AWS, Google Cloud, and Microsoft Azure offer various analytics services that efficiently handle large-scale data processing tasks.
Tools such as AWS Redshift, Google BigQuery, and Azure Synapse Analytics enable organisations to perform complex queries and generate insights at unprecedented speeds. These platforms support a variety of analytical workloads, from basic reporting to advanced machine learning models, empowering businesses to make data-driven decisions more effectively. - Scalability and flexibility
Cloud environments are designed for scalability. Unlike traditional on-premises systems, which often require significant capital investment to expand, cloud platforms provide a pay-as-you-go model that scales resources according to demand. This flexibility means businesses can effortlessly adjust their data storage and processing capabilities as their needs evolve. Whether you’re handling sudden spikes in data volume or experimenting with new analytics tools, the cloud’s elasticity ensures you have the resources you need when needed. - Enhanced data integration
Cloud migration facilitates better data integration across disparate sources. Modern cloud platforms come with robust data ingestion, transformation, and integration tools, which can unify various data streams into a single, coherent dataset. This integration capability is crucial for comprehensive analytics, as it allows firms to aggregate data from multiple sources, such as CRM systems, IoT devices, and transactional databases, into a unified platform. The result is a more complete and accurate view of business performance, leading to more insightful analysis.
Benefits of cloud-based data analytics
- Cost efficiency
One of the primary benefits of moving to the cloud is cost savings. Traditional data infrastructure requires significant upfront investment in hardware and ongoing expenses for maintenance and upgrades. In contrast, cloud platforms operate on a subscription or usage-based pricing model, which can be more economical. Organisations pay only for the resources they use and can scale up or down based on their needs. This model reduces capital expenditure and operational costs, making it easier for businesses to manage their budgets effectively. - Improved collaboration
Cloud-based analytics tools enhance collaboration by allowing multiple users to simultaneously access and work on the same datasets. This real-time data sharing fosters a collaborative environment where teams across different locations can contribute to data analysis and decision-making. Cloud platforms often include features such as shared dashboards and collaborative workspaces, which streamline communication and ensure that all stakeholders are working with the most up-to-date information. - Enhanced security and compliance
Cloud providers invest heavily in security measures to protect data. These measures include encryption, multi-factor authentication, and rigorous access controls. Additionally, cloud platforms are often compliant with various industry standards and regulations, such as GDPR, HIPAA, and SOC 2. This level of security and compliance is typically more advanced than what many organisations can achieve with on-premises solutions. By leveraging the cloud, businesses can enhance their data protection strategies and meet regulatory requirements more effectively.
Challenges in data migration to cloud
- Data quality and consistency
Migrating data to the cloud can uncover data quality and consistency issues. Discrepancies, duplicates, and incomplete records may become more apparent during the migration process. If not addressed, these issues can compromise the effectiveness of analytics and lead to inaccurate insights. Ensuring high data quality before migration is crucial for maintaining the integrity of analytical results. - Complexity of migration
Data migration involves several technical complexities, including data mapping, transformation, and validation. The process requires meticulous planning to ensure that data is transferred accurately and efficiently. Errors or oversights during migration can result in data loss or corruption, disrupting business operations and affecting analytical outcomes. - Integration with existing systems
Integrating cloud-based analytics solutions with existing on-premises systems or other cloud services can be challenging. Compatibility issues may arise, leading to potential disruptions in data flow and business processes. Developing a clear integration strategy and utilising middleware or API solutions can help address these challenges and ensure seamless system connectivity.
Mitigating migration challenges
- Comprehensive data assessment
Conducting a thorough assessment of your data before migration is essential. This involves identifying and addressing data quality issues, such as duplicates, inconsistencies, and inaccuracies. Implementing data cleansing and enrichment processes can help ensure that your data is in optimal condition before moving to the cloud. Additionally, creating a detailed data mapping plan will facilitate a smoother migration process and reduce the risk of errors. - Phased migration approach
Adopting a phased migration approach can help manage complexity and reduce risk. Begin with less critical data or applications and gradually move to more critical systems. This approach allows for adjustments and troubleshooting along the way, minimising potential disruptions to business operations. By migrating in stages, organisations can also gain insights into the migration process and refine their strategies as needed. - Leveraging migration tools and services
Utilise migration tools and services offered by cloud providers or third-party vendors to simplify the migration process. These tools often include features for automated data transfer, mapping, and validation, which can reduce manual effort and minimise errors. Additionally, working with experienced migration consultants or service providers can provide valuable expertise and support throughout the process. - Developing integration strategies
To address integration challenges, develop a comprehensive strategy outlining how cloud-based solutions will interact with existing systems. Utilise middleware, APIs, and integration platforms to ensure seamless connectivity and data flow between systems. Regularly test and validate integrations to identify and resolve issues before they impact business operations.
Future trends in cloud data analytics
- Integration of AI and machine learning
The integration of artificial intelligence (AI) and machine learning (ML) with cloud data analytics is set to grow. Cloud platforms increasingly incorporate AI and ML capabilities, which can enhance predictive analytics, automate insights, and identify patterns that may not be immediately apparent through traditional analysis. These technologies will enable businesses to make more informed, data-driven decisions and stay competitive in a rapidly evolving market. - Emergence of edge computing
Edge computing is gaining traction as a complement to cloud analytics. By processing data closer to its source, edge computing reduces latency and enables real-time data processing. This trend is particularly relevant for IoT applications and environments where immediate data insights are crucial. Combining edge computing and cloud analytics will provide a more robust and responsive data infrastructure. - Advances in data privacy technologies
As data privacy regulations become stricter, cloud providers are advancing privacy-enhancing technologies. Techniques such as federated learning, which allows models to be trained on decentralised data sources without exposing sensitive information, and advanced encryption methods will play a key role in ensuring data privacy and compliance. These innovations will help businesses navigate the evolving regulatory landscape while maintaining robust data protection.
Conclusion
Data migration to the cloud represents a significant opportunity for businesses to enhance their analytics capabilities. Organisations can unlock valuable insights and drive strategic decision-making by leveraging advanced analytics tools, benefiting from scalability and flexibility, and improving data integration. However, addressing challenges related to data quality, migration complexity, and system integration is essential for a successful transition. Looking ahead, the integration of AI and ML, the rise of edge computing, and advancements in data privacy technologies will shape the future of cloud-based analytics, offering even greater potential for innovation and growth. As businesses navigate this evolving landscape, staying informed about trends and best practices will be key to maximising cloud data migration's benefits.