-
Digital Natives
Unlock growth with Grant Thornton Bharat's Digital Natives solutions. Customised support for tech-driven companies in healthcare, gaming, and more.
-
Business Consulting
Our business consulting specialists offer a comprehensive blend of strategic advisory services. We assess the business, industry, operating model, synergy, skill sets and vision of the organisation and recommend the way forward
-
Digital Transformation Services
Grant Thornton’s digital transformation services help traditional businesses digitalise their business models with cloud technology, IoT consulting, app development and more DigiTech solutions.
-
Human Capital Consulting
Our Human Capital Consulting team harnesses technology and industry expertise to assist in constructing adaptable organisations with transparency, fostering productive and value-driven workforces, and inspiring employees to engage meaningfully in their tasks.
-
Production Linked Incentive Scheme
Production-linked Incentive Scheme by the Indian government is aimed at boosting manufacturing. Grant Thornton Bharat offers varied services across sectors to help businesses avail of this scheme.
-
Public Sector Advisory
Our Public Sector Advisory team has focused streams, aligned with the core priorities of the Government of India. We are responsible for providing innovative and customized technical and managerial solutions.
-
Tech Advisory
We have amalgamated Digital Transformation, IT Advisory & Information Management and Analytics into a new offering, DigiTech.
-
Direct Tax services
Our tax specialists offer a comprehensive blend of tax services, tax litigation, regulatory and compliance services, helping you navigate through complex business matters.
-
Indirect Tax Services
Get tax services by leading tax firm Grant Thornton India. Our indirect tax services include consulting, compliance and litigation services for corporate, international and transaction tax
-
Transfer pricing services
Our transfer pricing services experts provide a range of services from provision of APA services to handling large global assignments including Country by Country reporting.
-
US Tax
At Grant Thornton, we help individuals and dynamic companies deal with US tax laws, which are one of the most complicated tax legislations across the world.
-
Financial Services - Tax
Best financial advisory services, tailored for small and large businesses by the experts having comprehensive knowledge of domestic laws and access to multifaceted tools to provide a valuable results.
-
Financial Reporting consulting services
Our experts have significant hands-on experience in providing IFRS/US GAAP services, end-to-end solutions and support services to fulfil financial reporting requirements.
-
Fund accounting and financial reporting
International operations often lack standardisation and have varied local reporting formats and requirements. Our experts can offer proactive insights, practical guidance, and positive progress and help meet regulatory timeframes.
-
Compliance and Secretarial Services
Our experts can assist in overhauling the entire compliance machinery of the organisation through evaluation of the applicable statutory obligations, monitoring of adequate governance controls, reporting and providing ongoing support.
-
Global People Solutions
As businesses transcend borders, both domestic and global considerations need equal attention. Our interim CFO and financial controller support services help organisations meet the business vision.
-
Finance and accounting outsourcing
Our accounting experts assist organisations in managing their accounting and reporting. Our dedicated Integrated Knowledge and Capability Centre (IKCC), allows us to service both the domestic and global markets efficiently and cost-effectively
-
Compliance Management System
We have automation solutions for you that will allow meeting government requirements and remain diligent, which when failed, can lead to penalties and loss in revenue.
-
IKCC: Grant Thornton's Shared Service Centre
The India Knowledge and Capability Centre (IKCC), aimed at delivering solutions by developing capabilities, has completed four years of its journey.
-
Global compliance and reporting solutions
At Grant Thornton Bharat, we meet the challenges of our clients and help them unlock their potential for growth. Our professionals offer solutions tailored to meet our clients’ global accounting and statutory reporting requirements. With first-hand experience of local reporting requirements in more than 145+ locations worldwide, we provide seamless and consistent international service delivery through a single point of contact.
-
Related Party Transactions Governance
Grant Thornton Bharat's comprehensive related-party transaction services ensure good governance by adhering to regulatory requirements, promoting transparency, and providing robust policies for compliance, documentation, and accountability in related-party transactions.
-
Family Offices & Private Client Services
Grant Thornton Bharat Private Client Services offers tailored advisory for family-owned businesses, focusing on governance, compliance, tax, succession planning, and family office structuring to sustain wealth and preserve legacies across generations.
-
GTMitra: Tax & Regulatory Tool
GTMitra, a specialised tax and regulatory tool by Grant Thornton Bharat, supports multinational businesses in understanding laws and regulations for effective growth strategies.
-
Labour codes
Labour codes solutions help you transition through the new legislation. At Grant Thornton, we help businesses divide their approach to make sure a smooth transition.
-
Alerts
At Grant Thornton India, with the help of our tax alerts, we help to provide updates on how to minimise your tax exposure and risks.
-
Unlocking opportunities: India investment roadmap
The India Investment Roadmap resource is designed to navigate the complexities of Indian tax and regulatory laws, providing seamless guidance and a comprehensive set of solutions to ensure a smooth process for investors aiming to establish or expand their presence in India.
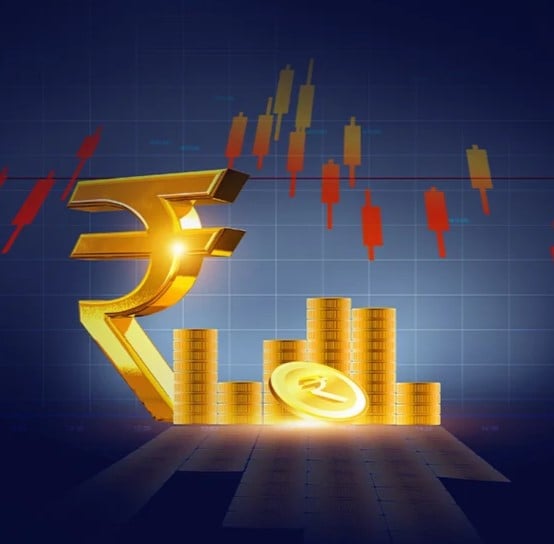
-
Cyber
In today’s time, businesses have gone through large transformation initiatives such as adoption of digital technologies, transition to cloud, use of advanced technologies et al.
-
Governance, Risk & Operations
Our Governance, Risk and Operations (GRO) services encompass Internal Audit, Enterprise Risk Management, Internal Financial Controls, IT advisory, Standard Operating Procedures and other services.
-
Risk analytics
Grant Thornton Bharat’s CLEARR Insights is a state-of-the art data analytics platform that will help you in seamless data analysis and efficient decision-making.
-
Forensic & Investigation Services
The team of forensic advisory services experts consists of the best intelligence corporate experts, and fraud risk, computer forensic experts to deliver most effective solutions to dynamic Indian businesses.
-
ESG consulting
Grant Thornton Bharat offers holistic ESG consulting solutions for sustainable business outcomes. With industry expertise and AI technology, we drive long-term value.
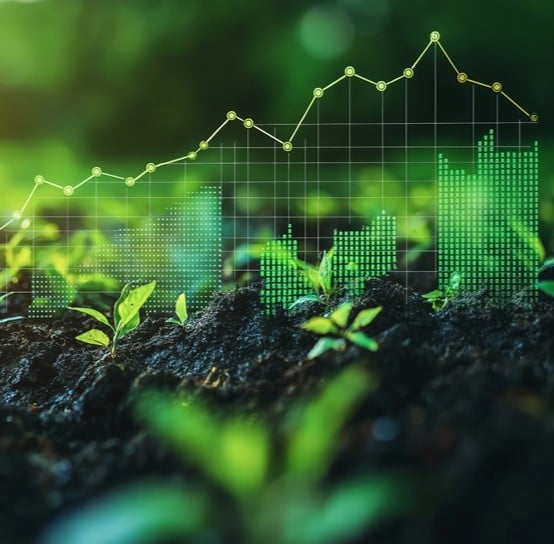
-
Transaction Tax Services
Our transaction tax experts understand your business, anticipate your needs and come up with robust tax solutions that help you achieve business objectives ensuring compliance and efficiency
-
Deal Advisory
Unlike other M&A advisory firm in India, we offer deal advisory services and work exclusively with controlled and well-designed strategies to help businesses grow, expand and create value.
-
Due Diligence
Grant Thornton’s financial due diligence services are aimed at corporate looking for mergers and acquisitions, private equity firms evaluating investments and businesses/promoters considering sale/divestment.
-
Valuations
As one of the leading valuation consultants in India, Grant Thornton specializes in all the aspects of the process like business valuation services, financial reporting, tax issues, etc.
-
Overseas Listing
Overseas listing presents a perfect platform for mid-sized Indian companies with global ambitions. Grant Thornton’s team of experts in listings, work closely with clients during all stages.
-
Debt & Special Situations Solutions
Grant Thornton Bharat offers specialist debt and special situations consulting services, including restructuring, insolvency, and asset tracing solutions.
-
Financial Reporting Advisory Services
Grant Thornton Bharat Financial Reporting Advisory Services offer end-to-end solutions for complex financial requirements, including GAAP conversions, IPO support, and hedge accounting advisory, ensuring accurate financial reporting and compliance.
-
Financial Statement Audit and Attestation Services
Grant Thornton Bharat offers customised financial statement audit and attestation services, ensuring impeccable quality and compliance with global standards. Our partner-led approach, technical expertise, and market credibility ensure effective solutions for your business needs.

- Agriculture
- Asset management
- Automotive and EV
- Aviation
- Banking
- Education and ed-tech
- Energy & Renewables
- Engineering & industrial products
- FinTech
- FMCG & consumer goods
- Food processing
- Gaming
- Healthcare
- Urban infrastructure
- Insurance
- Media
- Medical devices
- Metals & Mining
- NBFC
- Pharma, bio tech & life sciences
- Real estate and REITs
- Retail & E-commerce
- Specialty chemicals
- Sports
- Technology
- Telecom
- Transportation & logistics
- Tourism & hospitality
-
Thought leadership Co-lending in India: Expanding credit access for MSMEsIn today’s rapidly evolving financial landscape, co-lending has emerged as a key enabler of credit expansion in India, facilitating partnerships between banks and non-banking financial companies (NBFCs) to extend credit more efficiently to underserved segments.
-
Article Why India’s financial inclusion journey needs to focus on equity and access to creditFinancial services have expanded over a decade, giving millions access to bank accounts and digital payments. But true empowerment needs to reach every corner of the country if growth is to be long-term and sustained
-
Thought Leadership Competitive and sustainable agriculture & food processing in KeralaThe economy of Kerala is primarily driven by the services sector, which contributes 66% to the Gross State Domestic Product (GSDP).
-
Article Economic Survey 2024-25: Deregulation, investment and innovation for a Viksit BharatIndia's economic growth remains for a steady trajectory with real GDP expected to grow at 6.4% in FY25 and in the range of 6.3%-6.8% in FY26, reflecting resilience despite global uncertainties.
-
India-UK
India-UK
Introduction
AI has emerged as a transformative force for businesses across industries. Since the release of ChatGPT in December 2022, we’ve witnessed the rapid ascent of Gen AI, marking a pivotal moment in AI’s evolution. Many organizations believe AI could provide a significant competitive edge, with Gen AI potentially increasing global GDP by 3% to 7% over the next decade and adding trillions to the economy. This includes various subfields such as machine learning, natural language processing (NLP), computer vision (CV), robotics, and now Generative AI.
According to a 2024 CEO survey, 59% of CEOs believe AI will significantly impact their industries in the next three years, a sharp rise from 21% in 2023. Additionally, 87% of CEOs view AI’s benefits as outweighing its risks, making Generative AI and AI/ML services top tech priorities.
Artificial Intelligence (AI): Traditional AI vs. Generative AI
Artificial Intelligence services includes traditional techniques like Predictive AI and Causal AI. Predictive AI (or Analytical AI) uses algorithms to forecast future events based on historical data. Causal AI determines the causes and effects of events and is used in IT operations and AIOps.
Generative AI (Gen AI) services, leveraging foundational models such as large language models (LLMs), creates new content—text, code, images, audio, and more—by learning patterns from large datasets. This technology builds on existing data to generate novel outputs.
Gen AI use cases – 6C Framework
Gen AI might not be suitable for all use cases. It must be used complementary to Traditional or Discriminative AI. Our Gen AI use cases – 6C Framework – gives a broader direction for identifying use cases for Gen AI. According to our 6C Framework, its use cases fall under six broader categories.
Predictive AI
- Classification
- Regression
- Clustering
- Forecasting, Prediction
Causal AI
- Root cause analysis
- Anomaly detection
- Event corelation
Emergence of Hyper-modal AI (Gen AI + Predictive AI + Causal AI)
Hyper-modal AI combines Generative AI, Predictive AI, and Causal AI to address complex use cases. For example, in drug discovery, Predictive AI identifies potential drugs, while Causal AI analyses gene interactions to find the most effective treatment.
IT observability and operations tool maker Dynatrace recently announced their Hyper-model AI tool Davis. Historically, Davis has utilised Causal AI and Predictive AI for many AIOps use cases. Now they have augmented Davis with Generative AI capabilities, including the capability of interacting with the tool with natural language, Copilot AI Agent, and generating code for automation workflows.
Generative AI: Consume vs Customise vs Code Decision Framework
Gen AI Architecture Stack
Save
- Specialised infrastructure: Building and training Artificial Intelligence and Gen AI Models require specialised hardware and infrastructure, including GPUs, FPGA, fast and efficient networking (InfiniBand, Hollow core fibre/ HCF, RoCE / RDMA over Converged Ethernet, RDMA/ Remote Direct Memory Access), modern storage solutions, including cloud-based storage, and overall next-generation data centre technologies.
- Data and cloud: Success of your AI programmes would depend on your data strategy. Build a strong data foundation. Consider data as a product. Utilise modern data architecture patterns. Think about data lineage, data quality and data governance. The specialised infrastructure for AI can be easily accessed through a cloud service provider. Apart from infrastructure cloud service, providers also provide other services, including machine learning as a service, foundational model/ LLM as a service, data services, AI security, governance, RAI, etc.
- Foundational models: Foundational models power Gen AI. Models could be categorised by modality. Single modality models can generate only a single output type, e.g. either text or image. Multi-modality models can generate content multiple output type such as code, text, image, and video. Models also could be categorised by the number of parameters – Large language models (LLM) and small language models (SLM). Models also could be open source or closed source.
- Model customisation: Models need to be customised so that the model can understand your organisation, your client and your sector. This could be done in a number of ways, including prompt engineering, RAG (retrieval augmented generation), fine tuning and pre-training.
- Orchestrator: Our orchestration solutions integrate various AI capabilities to streamline operations and enhance efficiency.
- Gen AI apps: Gen AI powered apps include existing apps where new Gen AI powered features are added, such as newly built intelligent apps, AI agents and chatbots, digital assistants, etc.
AI ethics, security and governance – Responsible AI (RAI) and Explainable AI (XAI)
As AI systems and agents are being used in critical and impactful use cases from identifying the right candidate for a job to underwriting insurance to different healthcare and life sciences, use cases ensuring that these systems are unbiased, fair, safe, secure and reliable should be the topmost priority. These systems should not violate intellectual property (IP) and should abide by AI and data-related laws, regulations and compliances (e.g. the newly formed EU AI Act, Artificial Intelligence and Data Act (AIDA by Govt of Canada), GDPR, etc). Overall, AI systems should protect and reinforce positive human values.
Our responsible Artificial Intelligence services framework covers all aspects of AI ethics and governance. We utilise this framework in all our Gen AI and AI/ML projects.
Fairness and equality
Inclusivity & non-discrimination
Reliability and safety
Security and privacy
Intellectual property
Regulations & compliance
Transparency, explainability and interpretability
Protection and reinforcement of positive human values
Accountability
Governance
Scale your AI Initiatives – Our AI adoption framework
We can help you end-to-end, starting from AI readiness assessment to identifying and prioritising the optimum use cases for you to Gen AI POC to design and build. Our AI ethics and governance services include all aspects of Responsible AI, FinOps for AI workloads and Sustainable AI.
Our Gen AI and AI/ML services
We can help you end-to-end, starting from AI readiness assessment to identifying and prioritising the optimum use cases for you to Gen AI POC to design and build. Our AI ethics and governance services include all aspects of Responsible AI, FinOps for AI workloads and Sustainable AI.
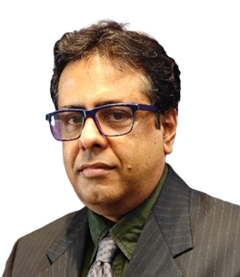