-
Digital Natives
Unlock growth with Grant Thornton Bharat's Digital Natives solutions. Customised support for tech-driven companies in healthcare, gaming, and more.
-
Business Consulting
Our business consulting specialists offer a comprehensive blend of strategic advisory services. We assess the business, industry, operating model, synergy, skill sets and vision of the organisation and recommend the way forward
-
Digital Transformation Services
Grant Thornton’s digital transformation services help traditional businesses digitalise their business models with cloud technology, IoT consulting, app development and more DigiTech solutions.
-
Human Capital Consulting
Our Human Capital Consulting team harnesses technology and industry expertise to assist in constructing adaptable organisations with transparency, fostering productive and value-driven workforces, and inspiring employees to engage meaningfully in their tasks.
-
Production Linked Incentive Scheme
Production-linked Incentive Scheme by the Indian government is aimed at boosting manufacturing. Grant Thornton Bharat offers varied services across sectors to help businesses avail of this scheme.
-
Public Sector Advisory
Our Public Sector Advisory team has focused streams, aligned with the core priorities of the Government of India. We are responsible for providing innovative and customized technical and managerial solutions.
-
Tech Advisory
We have amalgamated Digital Transformation, IT Advisory & Information Management and Analytics into a new offering, DigiTech.
-
Direct Tax services
Our tax specialists offer a comprehensive blend of tax services, tax litigation, regulatory and compliance services, helping you navigate through complex business matters.
-
Indirect Tax Services
Get tax services by leading tax firm Grant Thornton India. Our indirect tax services include consulting, compliance and litigation services for corporate, international and transaction tax
-
Transfer pricing services
Our transfer pricing services experts provide a range of services from provision of APA services to handling large global assignments including Country by Country reporting.
-
US Tax
At Grant Thornton, we help individuals and dynamic companies deal with US tax laws, which are one of the most complicated tax legislations across the world.
-
Financial Services - Tax
Best financial advisory services, tailored for small and large businesses by the experts having comprehensive knowledge of domestic laws and access to multifaceted tools to provide a valuable results.
-
Financial Reporting consulting services
Our experts have significant hands-on experience in providing IFRS/US GAAP services, end-to-end solutions and support services to fulfil financial reporting requirements.
-
Fund accounting and financial reporting
International operations often lack standardisation and have varied local reporting formats and requirements. Our experts can offer proactive insights, practical guidance, and positive progress and help meet regulatory timeframes.
-
Compliance and Secretarial Services
Our experts can assist in overhauling the entire compliance machinery of the organisation through evaluation of the applicable statutory obligations, monitoring of adequate governance controls, reporting and providing ongoing support.
-
Global People Solutions
As businesses transcend borders, both domestic and global considerations need equal attention. Our interim CFO and financial controller support services help organisations meet the business vision.
-
Finance and accounting outsourcing
Our accounting experts assist organisations in managing their accounting and reporting. Our dedicated Integrated Knowledge and Capability Centre (IKCC), allows us to service both the domestic and global markets efficiently and cost-effectively
-
Compliance Management System
We have automation solutions for you that will allow meeting government requirements and remain diligent, which when failed, can lead to penalties and loss in revenue.
-
IKCC: Grant Thornton's Shared Service Centre
The India Knowledge and Capability Centre (IKCC), aimed at delivering solutions by developing capabilities, has completed four years of its journey.
-
Global compliance and reporting solutions
At Grant Thornton Bharat, we meet the challenges of our clients and help them unlock their potential for growth. Our professionals offer solutions tailored to meet our clients’ global accounting and statutory reporting requirements. With first-hand experience of local reporting requirements in more than 145+ locations worldwide, we provide seamless and consistent international service delivery through a single point of contact.
-
Related Party Transactions Governance
Grant Thornton Bharat's comprehensive related-party transaction services ensure good governance by adhering to regulatory requirements, promoting transparency, and providing robust policies for compliance, documentation, and accountability in related-party transactions.
-
Family Offices & Private Client Services
Grant Thornton Bharat Private Client Services offers tailored advisory for family-owned businesses, focusing on governance, compliance, tax, succession planning, and family office structuring to sustain wealth and preserve legacies across generations.
-
GTMitra: Tax & Regulatory Tool
GTMitra, a specialised tax and regulatory tool by Grant Thornton Bharat, supports multinational businesses in understanding laws and regulations for effective growth strategies.
-
Labour codes
Labour codes solutions help you transition through the new legislation. At Grant Thornton, we help businesses divide their approach to make sure a smooth transition.
-
Alerts
At Grant Thornton India, with the help of our tax alerts, we help to provide updates on how to minimise your tax exposure and risks.
-
Unlocking opportunities: India investment roadmap
The India Investment Roadmap resource is designed to navigate the complexities of Indian tax and regulatory laws, providing seamless guidance and a comprehensive set of solutions to ensure a smooth process for investors aiming to establish or expand their presence in India.
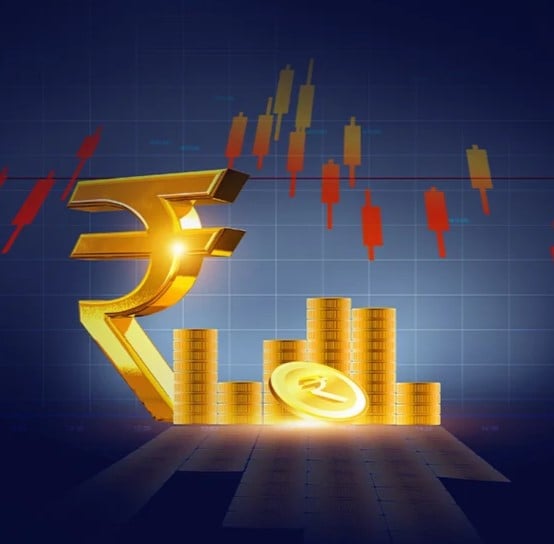
-
Cyber
In today’s time, businesses have gone through large transformation initiatives such as adoption of digital technologies, transition to cloud, use of advanced technologies et al.
-
Governance, Risk & Operations
Our Governance, Risk and Operations (GRO) services encompass Internal Audit, Enterprise Risk Management, Internal Financial Controls, IT advisory, Standard Operating Procedures and other services.
-
Risk analytics
Grant Thornton Bharat’s CLEARR Insights is a state-of-the art data analytics platform that will help you in seamless data analysis and efficient decision-making.
-
Forensic & Investigation Services
The team of forensic advisory services experts consists of the best intelligence corporate experts, and fraud risk, computer forensic experts to deliver most effective solutions to dynamic Indian businesses.
-
ESG consulting
Grant Thornton Bharat offers holistic ESG consulting solutions for sustainable business outcomes. With industry expertise and AI technology, we drive long-term value.
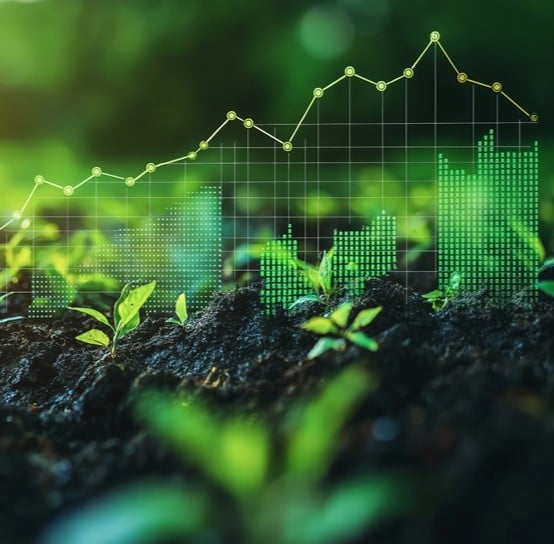
-
Transaction Tax Services
Our transaction tax experts understand your business, anticipate your needs and come up with robust tax solutions that help you achieve business objectives ensuring compliance and efficiency
-
Deal Advisory
Unlike other M&A advisory firm in India, we offer deal advisory services and work exclusively with controlled and well-designed strategies to help businesses grow, expand and create value.
-
Due Diligence
Grant Thornton’s financial due diligence services are aimed at corporate looking for mergers and acquisitions, private equity firms evaluating investments and businesses/promoters considering sale/divestment.
-
Valuations
As one of the leading valuation consultants in India, Grant Thornton specializes in all the aspects of the process like business valuation services, financial reporting, tax issues, etc.
-
Overseas Listing
Overseas listing presents a perfect platform for mid-sized Indian companies with global ambitions. Grant Thornton’s team of experts in listings, work closely with clients during all stages.
-
Debt & Special Situations Solutions
Grant Thornton Bharat offers specialist debt and special situations consulting services, including restructuring, insolvency, and asset tracing solutions.
-
Financial Reporting Advisory Services
Grant Thornton Bharat Financial Reporting Advisory Services offer end-to-end solutions for complex financial requirements, including GAAP conversions, IPO support, and hedge accounting advisory, ensuring accurate financial reporting and compliance.
-
Financial Statement Audit and Attestation Services
Grant Thornton Bharat offers customised financial statement audit and attestation services, ensuring impeccable quality and compliance with global standards. Our partner-led approach, technical expertise, and market credibility ensure effective solutions for your business needs.

- Agriculture
- Asset management
- Automotive and EV
- Aviation
- Banking
- Education and ed-tech
- Energy & Renewables
- Engineering & industrial products
- FinTech
- FMCG & consumer goods
- Food processing
- Gaming
- Healthcare
- Urban infrastructure
- Insurance
- Media
- Medical devices
- Metals & Mining
- NBFC
- Pharma, bio tech & life sciences
- Real estate and REITs
- Retail & E-commerce
- Specialty chemicals
- Sports
- Technology
- Telecom
- Transportation & logistics
- Tourism & hospitality
-
Thought leadership Co-lending in India: Expanding credit access for MSMEsIn today’s rapidly evolving financial landscape, co-lending has emerged as a key enabler of credit expansion in India, facilitating partnerships between banks and non-banking financial companies (NBFCs) to extend credit more efficiently to underserved segments.
-
Article Why India’s financial inclusion journey needs to focus on equity and access to creditFinancial services have expanded over a decade, giving millions access to bank accounts and digital payments. But true empowerment needs to reach every corner of the country if growth is to be long-term and sustained
-
Thought Leadership Competitive and sustainable agriculture & food processing in KeralaThe economy of Kerala is primarily driven by the services sector, which contributes 66% to the Gross State Domestic Product (GSDP).
-
Article Economic Survey 2024-25: Deregulation, investment and innovation for a Viksit BharatIndia's economic growth remains for a steady trajectory with real GDP expected to grow at 6.4% in FY25 and in the range of 6.3%-6.8% in FY26, reflecting resilience despite global uncertainties.
-
India-UK
India-UK
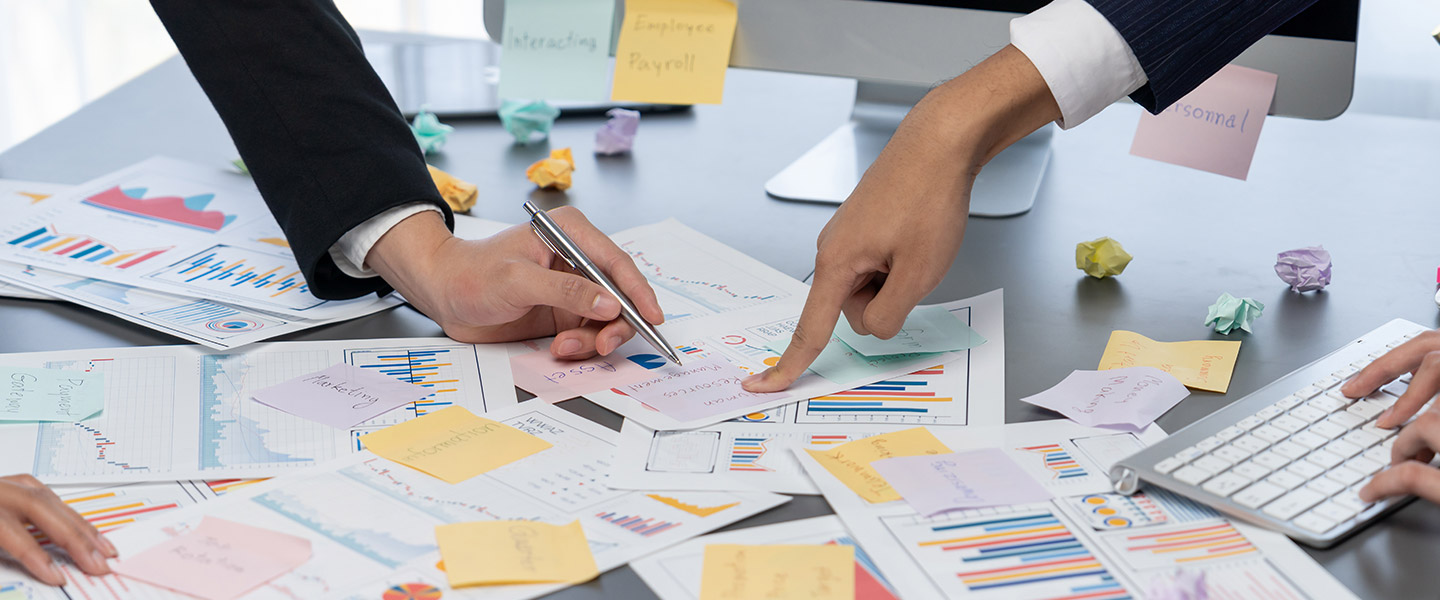
In today’s world, data is extensively used in any business, acting as the fuel to drive decision-making, innovation, as well as shape strategic initiatives. Whether it is customer demographics, sales figures, market trends, risk parameters or operational metrics, businesses rely on data to gain insights, identify opportunities, and mitigate risks. However, its value is only as good as its quality. Inaccurate, incomplete or irrelevant data can lead to misguided decisions, missed opportunities, and inefficiencies. Therefore, ensuring data quality is paramount for organisations aiming to thrive in today's competitive landscape.
In this blog, we have attempted to delve into the intricacies of data quality and address common challenges in data management. Moreover, we will outline best practices for establishing robust metrics and key performance indicators (KPIs) that enable organisations to measure and maintain the quality of their data effectively.
Understanding data quality
Data quality refers to the reliability, accuracy, completeness and relevance of information for its intended purpose. High-quality data needs to be dependable, consistent, complete and aligned to the organisation’s business objectives. Yet, achieving and maintaining such standards pose significant hurdles. These include:
Inaccuracies: Data inaccuracies stem from various sources, including human error, system glitches, or outdated information. These inaccuracies can lead to misguided decisions and erode trust in data-driven insights.
Inconsistencies: Divergent formats, duplicate entries, and conflicting records lead to data inconsistencies, hindering seamless analysis and integration across systems.
Incompleteness: Missing or incomplete data paints an incomplete picture, making it difficult to derive meaningful conclusions and insights for appropriate decision-making.
Irrelevance: Not all data is valuable if it is not relevant. Irrelevant or outdated information clutters databases, impeding efficiency and obscuring actionable insights.
Addressing common challenges in data quality management
Effective data quality management can help guide organisations toward cleaner and more reliable data. Yet, this process is fraught with multiple challenges:
Data profiling: Conducting comprehensive data profiling enables organisations to assess the quality of their datasets, identifying anomalies, outliers and inconsistencies
Data cleansing: Implementing robust data cleansing procedures involves detecting and rectifying errors, duplicates, incompleteness and inconsistencies to maintain data integrity
Data governance: Establishing clear frameworks ensures accountability, transparency, and compliance across the data lifecycle, from collection, ingestion to disposal
Continuous monitoring: Embracing real-time monitoring tools and techniques enables proactive identification and resolution of data quality issues before they escalate. Identifying critical data elements (CDE) and ensuring data quality both on proactive (strategic) and reactive (incident management) basis will ensure reliable data for decision-making through actionable insights and advanced analytics.
Best practices to establish data quality metrics and KPIs
Establishing meaningful metrics and KPIs is pivotal to gauge the efficacy of data quality initiatives. Here are some of the best practices (not exhaustive) to consider:
Align with business objectives: Align metrics directly with organisational goals and priorities, focusing on areas critical to driving value and performance
Quantify quality dimensions: Define metrics that quantify key dimensions of data quality, including accuracy, completeness, consistency, timeliness, and relevancy
Set clear benchmarks: Establish clear benchmarks and thresholds after baselining for each metric, delineating acceptable levels of data quality and highlighting areas requiring improvement
Monitor continuously: Implement real-time monitoring mechanisms to track data quality metrics continuously, enabling prompt detection of deviations or anomalies
Iterate and improve: Embrace a culture of continuous improvement, iterating on data quality metrics based on feedback, insights, goals and evolving business needs
Collaborate across functions: Foster collaboration between IT, data management, and business stakeholders to ensure alignment of data quality metrics with operational objectives and user requirements
In conclusion, the indispensable role of data in modern business operations (both frontend and backend) cannot be ignored. From strategic decision-making through actionable insights to driving operational efficiencies, data serves as the cornerstone upon which organisational success is built.
In today's dynamic and fiercely competitive landscape, businesses that prioritise data quality are expected to gain a strategic advantage, leveraging their information assets to generate insights, enable innovation, enhance customer experiences, mitigate risks and achieve sustainable growth. By embracing a culture of continuous improvement and collaboration, organisations can navigate the complexities of the data landscape with confidence and clarity, propelling themselves towards long-term success.