Grant Thornton offers best business advisory services for foreign and Indian companies. If you also want to do business in India, contact us!
Adapt to dynamic markets with tailored solutions and expert business consultancy.
-
Digital Natives
Unlock growth with Grant Thornton Bharat's Digital Natives solutions. Customised support for tech-driven companies in healthcare, gaming, and more.
-
Business Consulting
Our business consulting specialists offer a comprehensive blend of strategic advisory services. We assess the business, industry, operating model, synergy, skill sets and vision of the organisation and recommend the way forward
-
Digital Transformation Services
Grant Thornton’s digital transformation services help traditional businesses digitalise their business models with cloud technology, IoT consulting, app development and more DigiTech solutions.
-
Human Capital Consulting
Our Human Capital Consulting team harnesses technology and industry expertise to assist in constructing adaptable organisations with transparency, fostering productive and value-driven workforces, and inspiring employees to engage meaningfully in their tasks.
-
Production Linked Incentive Scheme
Production-linked Incentive Scheme by the Indian government is aimed at boosting manufacturing. Grant Thornton Bharat offers varied services across sectors to help businesses avail of this scheme.
-
Public Sector Advisory
Our Public Sector Advisory team has focused streams, aligned with the core priorities of the Government of India. We are responsible for providing innovative and customized technical and managerial solutions.
-
Tech Advisory
We have amalgamated Digital Transformation, IT Advisory & Information Management and Analytics into a new offering, DigiTech.
Tax advisory and advisory services in India by Grant Thornton aim to help businesses of all sizes to formulate and implement effective strategies to optimize...
-
Direct Tax services
Our tax specialists offer a comprehensive blend of tax services, tax litigation, regulatory and compliance services, helping you navigate through complex business matters.
-
Indirect Tax Services
Get tax services by leading tax firm Grant Thornton India. Our indirect tax services include consulting, compliance and litigation services for corporate, international and transaction tax
-
Transfer pricing services
Our transfer pricing services experts provide a range of services from provision of APA services to handling large global assignments including Country by Country reporting.
-
US Tax
At Grant Thornton, we help individuals and dynamic companies deal with US tax laws, which are one of the most complicated tax legislations across the world.
-
Financial Services - Tax
Best financial advisory services, tailored for small and large businesses by the experts having comprehensive knowledge of domestic laws and access to multifaceted tools to provide a valuable results.
-
Financial Reporting consulting services
Our experts have significant hands-on experience in providing IFRS/US GAAP services, end-to-end solutions and support services to fulfil financial reporting requirements.
-
Fund accounting and financial reporting
International operations often lack standardisation and have varied local reporting formats and requirements. Our experts can offer proactive insights, practical guidance, and positive progress and help meet regulatory timeframes.
-
Compliance and Secretarial Services
Our experts can assist in overhauling the entire compliance machinery of the organisation through evaluation of the applicable statutory obligations, monitoring of adequate governance controls, reporting and providing ongoing support.
-
Global People Solutions
As businesses transcend borders, both domestic and global considerations need equal attention. Our interim CFO and financial controller support services help organisations meet the business vision.
-
Finance and accounting outsourcing
Our accounting experts assist organisations in managing their accounting and reporting. Our dedicated Integrated Knowledge and Capability Centre (IKCC), allows us to service both the domestic and global markets efficiently and cost-effectively
-
Compliance Management System
We have automation solutions for you that will allow meeting government requirements and remain diligent, which when failed, can lead to penalties and loss in revenue.
-
IKCC: Grant Thornton's Shared Service Centre
The India Knowledge and Capability Centre (IKCC), aimed at delivering solutions by developing capabilities, has completed four years of its journey.
-
Global compliance and reporting solutions
At Grant Thornton Bharat, we meet the challenges of our clients and help them unlock their potential for growth. Our professionals offer solutions tailored to meet our clients’ global accounting and statutory reporting requirements. With first-hand experience of local reporting requirements in more than 145+ locations worldwide, we provide seamless and consistent international service delivery through a single point of contact.
-
Related Party Transactions Governance
Grant Thornton Bharat's comprehensive related-party transaction services ensure good governance by adhering to regulatory requirements, promoting transparency, and providing robust policies for compliance, documentation, and accountability in related-party transactions.
-
Family Offices & Private Client Services
Grant Thornton Bharat Private Client Services offers tailored advisory for family-owned businesses, focusing on governance, compliance, tax, succession planning, and family office structuring to sustain wealth and preserve legacies across generations.
-
GTMitra: Tax & Regulatory Tool
GTMitra, a specialised tax and regulatory tool by Grant Thornton Bharat, supports multinational businesses in understanding laws and regulations for effective growth strategies.
-
Labour codes
Labour codes solutions help you transition through the new legislation. At Grant Thornton, we help businesses divide their approach to make sure a smooth transition.
-
Alerts
At Grant Thornton India, with the help of our tax alerts, we help to provide updates on how to minimise your tax exposure and risks.
-
Unlocking opportunities: India investment roadmap
The India Investment Roadmap resource is designed to navigate the complexities of Indian tax and regulatory laws, providing seamless guidance and a comprehensive set of solutions to ensure a smooth process for investors aiming to establish or expand their presence in India.
Related insights:
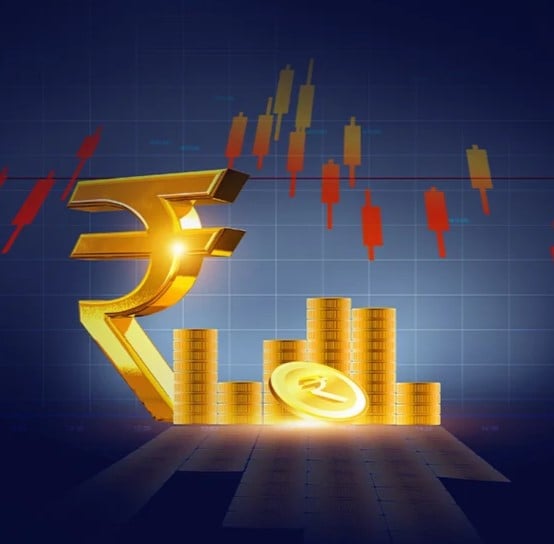
Grant Thornton Bharat ESG and Risk Consulting provides expert governance, IT security, and forensic services, ensuring robust processes and controls for...
-
Cyber
In today’s time, businesses have gone through large transformation initiatives such as adoption of digital technologies, transition to cloud, use of advanced technologies et al.
-
Governance, Risk & Operations
Our Governance, Risk and Operations (GRO) services encompass Internal Audit, Enterprise Risk Management, Internal Financial Controls, IT advisory, Standard Operating Procedures and other services.
-
Risk analytics
Grant Thornton Bharat’s CLEARR Insights is a state-of-the art data analytics platform that will help you in seamless data analysis and efficient decision-making.
-
Forensic & Investigation Services
The team of forensic advisory services experts consists of the best intelligence corporate experts, and fraud risk, computer forensic experts to deliver most effective solutions to dynamic Indian businesses.
-
ESG consulting
Grant Thornton Bharat offers holistic ESG consulting solutions for sustainable business outcomes. With industry expertise and AI technology, we drive long-term value.
Related insights:
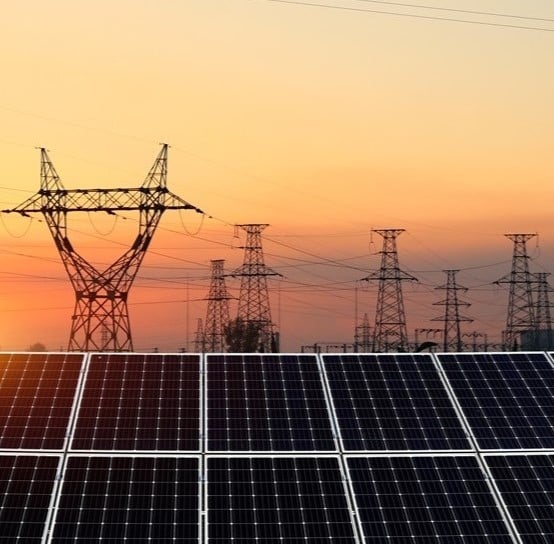
Budget 2025
Budget 2025: A deep dive into the measures for the Energy Sector
The Indian energy sector stands at the cusp of a transformative leap, driven by a robust policy framework and an ambitious vision for energy security, sustainability, and affordability.
Supporting you to grow your business profitably through our partner-led deals service.
-
Transaction Tax Services
Our transaction tax experts understand your business, anticipate your needs and come up with robust tax solutions that help you achieve business objectives ensuring compliance and efficiency
-
Deal Advisory
Unlike other M&A advisory firm in India, we offer deal advisory services and work exclusively with controlled and well-designed strategies to help businesses grow, expand and create value.
-
Due Diligence
Grant Thornton’s financial due diligence services are aimed at corporate looking for mergers and acquisitions, private equity firms evaluating investments and businesses/promoters considering sale/divestment.
-
Valuations
As one of the leading valuation consultants in India, Grant Thornton specializes in all the aspects of the process like business valuation services, financial reporting, tax issues, etc.
-
Overseas Listing
Overseas listing presents a perfect platform for mid-sized Indian companies with global ambitions. Grant Thornton’s team of experts in listings, work closely with clients during all stages.
-
Debt & Special Situations Solutions
Grant Thornton Bharat offers specialist debt and special situations consulting services, including restructuring, insolvency, and asset tracing solutions.
Grant Thornton Bharat offers tailored assurance and auditing services in India, ensuring NFRA compliance, sector expertise, and risk-based audits for credible...
-
Financial Reporting Advisory Services
Grant Thornton Bharat Financial Reporting Advisory Services offer end-to-end solutions for complex financial requirements, including GAAP conversions, IPO support, and hedge accounting advisory, ensuring accurate financial reporting and compliance.
-
Financial Statement Audit and Attestation Services
Grant Thornton Bharat offers customised financial statement audit and attestation services, ensuring impeccable quality and compliance with global standards. Our partner-led approach, technical expertise, and market credibility ensure effective solutions for your business needs.
Related insights:

Thought leadership
Quarterly GAAP Bulletin – January 2025
A summary of significant accounting, auditing and related updates
Grant Thornton’s Global Delivery Services works to provide innovative multi-disciplinary solutions across service lines and expertise areas, with a global...
Industries
- Agriculture
- Asset management
- Automotive and EV
- Aviation
- Banking
- Education and ed-tech
- Energy & Renewables
- Engineering & industrial products
- FinTech
- FMCG & consumer goods
- Food processing
- Gaming
- Healthcare
- Urban infrastructure
- Insurance
- Media
- Medical devices
- Metals & Mining
- NBFC
- Pharma, bio tech & life sciences
- Real estate and REITs
- Retail & E-commerce
- Specialty chemicals
- Sports
- Technology
- Telecom
- Transportation & logistics
- Tourism & hospitality
Helping you shape the future of your industry
We offer top-tier agriculture consulting services, tackling challenges and driving growth in India's agri-industry. Discover our expert solutions now.
Related insights:
-
Article Economic Survey 2024-25: Deregulation, investment and innovation for a Viksit BharatIndia's economic growth remains for a steady trajectory with real GDP expected to grow at 6.4% in FY25 and in the range of 6.3%-6.8% in FY26, reflecting resilience despite global uncertainties.
-
Article Navigating market volatility and government support for Indian agricultureIndia's agricultural sector, the backbone of the nation's economy, contributes ~17% to the GDP and employs over half of the workforce.
-
Article Improving credit penetration in BiharRBI’s priority sector lending guidelines have a key role to play in providing credit to small farmers, artisans and micro firms
-
Article Sustainable farming and its economic imperativeSustainable farming in India is crucial for food security, economic growth, and environmental conservation, requiring government support and farmer education.
We delivers tailored asset management consulting, from governance to technology transformation, enhancing growth and compliance.
We offers expert automotive consulting for building better mobility businesses, navigating EV transitions, and staying ahead globally.
Elevate your aviation business with expert consulting services. From strategy to implementation, we guide you towards success in a dynamic industry.
We offers expert banking consulting services, including regulatory compliance, risk management, governance, and transformation solutions.
Elevate your education sector with expert consulting services. Navigate challenges, innovate, and achieve excellence with our guidance.
Elevate your energy sector with our expert renewable consulting services. We guide you towards sustainable solutions for a brighter future.
Our engineering consulting empowers firms with digitization, strategy, and market insights for sustainable growth.
We specialize in fintech consulting services, offering tailored solutions for industry challenges like risk management, regulatory compliance, and finance...
Explore our consumer goods consulting services, covering direct-to-consumer strategies, supply chain optimization, and shop floor transformation.
Elevate your food processing industry with expert consulting. Unlock growth, minimize waste, and drive economic prosperity for India's future.
Unlock new levels of success in the gaming industry with Grant Thornton Bharat. Expert gaming consulting, regulatory compliance, tax advisory, and deals...
Grant Thornton Bharat healthcare consulting experts facilitating strategic planning, operational execution, and regulatory compliance for sustainable growth.
We offers infrastructure consulting to help cities build competitive advantage, mitigate risks, improve efficiency, and create flexible organizations.
Our experts provides comprehensive insurance consulting services, addressing complex challenges and opportunities in the evolving insurance landscape.
Our team of media and entertainment consulting experts crafts strategies and capabilities, empowering companies to adapt to today's industry trends.
Unlock growth potential in India's medical device market with Grant Thornton Bharat expert consulting. Navigate regulations and innovate for success.
We offers metals & mining consulting services to firms with digital integration, collaborative strategies & data-driven insights for growth.
Grant Thornton Bharat offers tailored strategic guidance, robust risk management, and innovative solutions for NBFCs, enhancing performance and compliance.
Navigate towards sustainable success in the health-outcomes-driven ecosystem with our Life Sciences consulting services.
Unlock growth opportunities by turning critical real estate issues into success with Grant thornton bharat expert real estate consulting services.
Unlock bold visions for your online and e-commerce channels with Grant Thornton Bharat digital expertise and strategic guidance. Explore now.
We offers specialized chemical consulting services, leveraging advanced analytics for informed decisions. Enhance your industry advantage now!
We provide sports consulting services that help organisations and governments, regardless of size, enhance their value through strategic planning, business...
Elevate your business with custom tech solutions. Navigate challenges & seize growth opportunities with Grant Thornton Bharat technology consulting services.
Unlock growth potential in India's booming telecom market with Grant Thornton Bharat strategic consulting services. Trusted partners across the value chain.
We offers innovative transportation solutions, navigating industry changes for impactful results in a dynamic business landscape.
Grant Thornton Bharat offers tourism consulting services, helping clients achieve competitive advantage in India's dynamic tourism and hospitality industry.
Our global presence
Are you seeking global opportunities? Whether it's establishing operations, enhancing capacities, or making acquisitions, we're here to help.
-
India-UK
India-UK
Helping Global Indians with cross-border investment, expansion and mobility.